Welcome to Diffusion Reinforcement Learning
Diffusion Reinforcement Learning (DRL) is an advanced approach that integrates the principles of diffusion processes with reinforcement learning algorithms. This hybrid method leverages the strength of diffusion models, which are adept at generating high-quality, continuous data representations, with the strategic decision-making capabilities of reinforcement learning. DRL is particularly effective in environments where the agent’s actions can be seen as influencing or navigating through a continuous state space, allowing for more nuanced and precise control strategies. By combining these two methodologies, DRL enhances the agent’s ability to learn optimal policies in complex, dynamic environments, making it a powerful tool for tasks that require sophisticated, long-term planning and execution.
Representative Publications
Changyuan Zhao, Hongyang Du, Dusit Niyato, Jiawen Kang, Zehui Xiong, Dong In Kim, Xuemin (Sherman) Shen, Khaled B. Letaief
Under Review
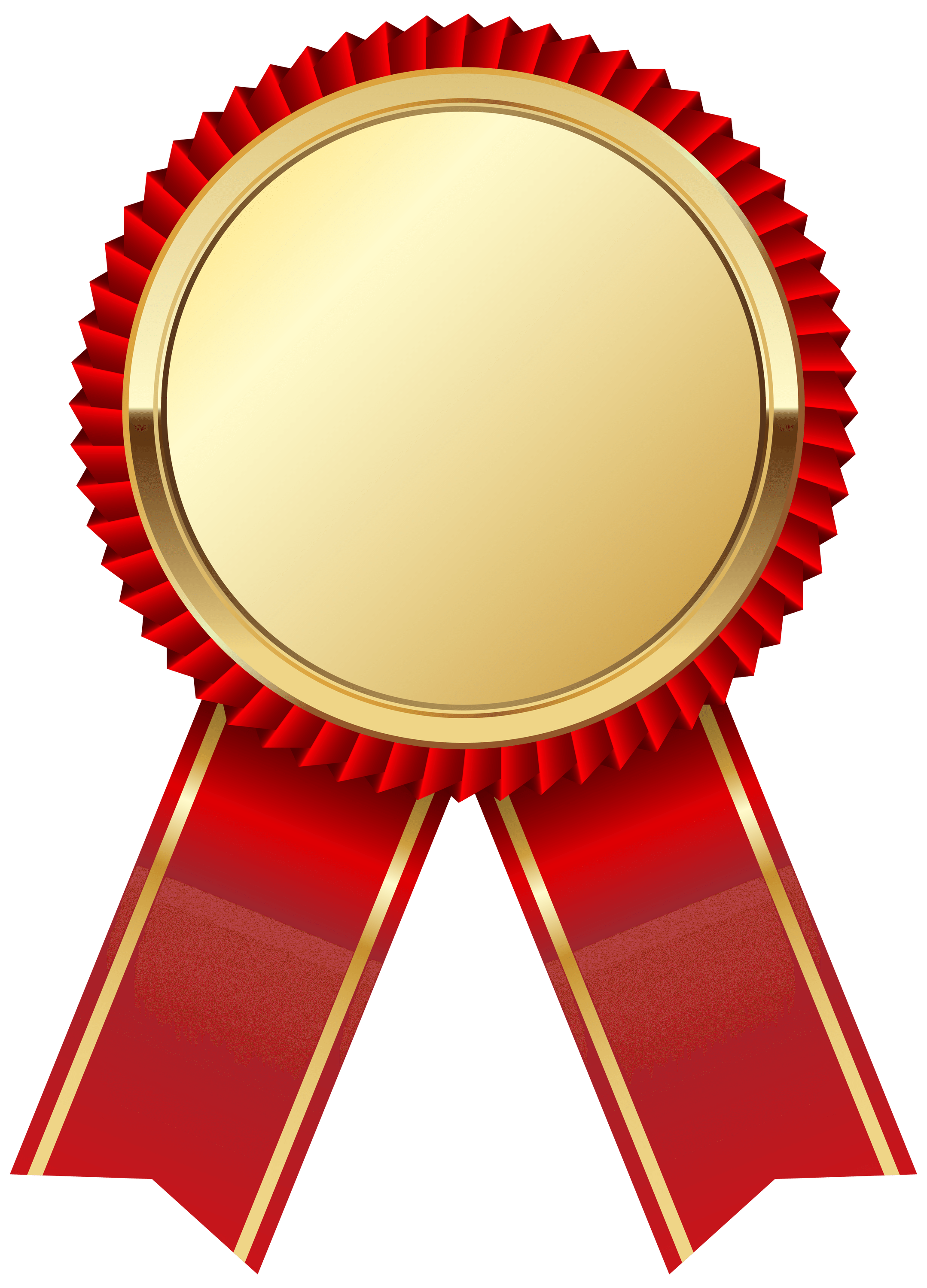

Yinqiu Liu, Ruichen Zhang, Hongyang Du, Dusit Niyato, Jiawen Kang, Zehui Xiong, and Dong In Kim
Under Review
Hongyang Du, Ruichen Zhang, Yinqiu Liu, Jiacheng Wang, Yijing Lin, Zonghang Li, Dusit Niyato, Jiawen Kang, Zehui Xiong, Shuguang Cui, Bo Ai, Haibo Zhou, Dong In Kim
IEEE COMST
Hongyang Du, Ruichen Zhang, Dusit Niyato, Jiawen Kang, Zehui Xiong, Shuguang Cui, Xuemin Shen, Dong In Kim
Under Review
Hongyang Du, Zonghang Li, Dusit Niyato, Jiawen Kang, Zehui Xiong, Huawei Huang, and Shiwen Mao
IEEE Transactions on Mobile Computing
Xumin Huang, Peichun Li, Hongyang Du, Jiawen Kang, Dusit Niyato, Dong In Kim, Yuan Wu
IEEE Network Magazine
Yinqiu Liu, Hongyang Du, Dusit Niyato, Jiawen Kang, Zehui Xiong, Dong In Kim, Abbas Jamalipour
IEEE Network Magazine